Modern medicine has achieved remarkable advancements, offering treatments and cures previously unimaginable. Consider implantable defibrillators that regulate heart rhythms, reducing the risk of cardiac arrest. These breakthroughs would not have been possible without the rigorous research of clinical trials, which evaluate the effects of medical interventions on human participants.
Unfortunately, the clinical trial process has become slower and more expensive. Only one in seven drugs that enter phase I trials, the first stage of safety testing, are eventually approved. Bringing a new medicinal product to market currently requires nearly a billion dollars and a decade of work, with half of that time and money spent on clinical trials. These trials face numerous hurdles, including recruitment inefficiencies, limited diversity, and patient inaccessibility, all of which slow drug discovery and increase costs. Fortunately, recent advancements in Artificial Intelligence (AI) have the potential to break this trend and transform drug development for the better.
From AI models that predict complex protein interactions to AI-powered lab assistants streamlining routine tasks, AI-driven innovation is reshaping the pharmaceutical landscape. Adopting new AI capabilities to address clinical trial barriers can enhance the trial process for patients, physicians, and BioPharma, paving the way for new impactful drugs and, potentially, better health outcomes.
Barriers to Drug Development
Drugs in development face significant challenges during clinical trials, which contributes to the alarmingly low approval rates from regulatory bodies like the U.S. Food and Drug Administration (FDA). As a result, many promising investigational medicines never reach the market. Key challenges include trial design setbacks, low patient recruitment, and limited patient accessibility and diversity—issues that compound one another and hinder progress and equity in drug development.
1. Trial Site Selection Challenges
The success of a clinical trial depends greatly on trial sites recruiting and enrolling a sufficient number of eligible participants. Site selection traditionally relies on a variety of factors, including historical performance in previous trials, local patient demographics, research capabilities and infrastructure, and more. Gathering and assessing the data for each factor is complex, and the results may not always accurately indicate whether a site is appropriate for a trial. Data can be outdated or incomplete, especially if based on a limited number of studies.
Data for site selection comes from diverse sources, such as internal databases, subscription services, vendors, and Contract Research Organizations. Aggregating and assessing this information can be confusing, which can lead to suboptimal decisions on trial sites. As a result, sponsors may overestimate or underestimate their ability to recruit patients, leading to wasted resources, delays, and low retention rates.
How can AI help? By training AI models with historical and real-time data from potential sites, trial sponsors can predict patient enrollment rates and a site’s performance, optimizing site allocation, reducing over- or under-enrollment, and improving overall efficiency and cost. These models can also rank potential sites by identifying the best combination of attributes and factors that align with study objectives and recruitment strategies.
AI models trained with clinical trial metadata, medical and pharmacy claims data, and patient data from membership services can also help identify clinical trial sites that provide access to diverse, relevant patient populations. Sites can be centrally located for underrepresented groups or even take place in popular community locations, helping to address both patient accessibility and lack of diversity.
2. Low Patient Recruitment
Patient recruitment remains a major bottleneck in clinical trials, consuming up to one-third of a study’s duration. One in five trials fail to recruit the required number of participants. As trials become more complex—with additional patient touchpoints, stricter inclusion and exclusion criteria, and increasingly sophisticated study designs—recruitment challenges continue to grow. Research links increasing protocol complexity with declining patient enrollment and retention rates.
Strict eligibility criteria, designed to ensure participant safety and study integrity, often limit access to treatment and disproportionately exclude certain patient populations, including older adults and racial, ethnic, and gender minorities. In oncology trials, an estimated 17–21% of patients cannot enroll due to these restrictions.
AI has the potential to optimize patient eligibility criteria and recruitment. Recruitment has traditionally required physicians to manually screen patients, which is incredibly time-consuming. AI can efficiently and effectively match patient profiles against suitable trials.
For example, machine learning algorithms can automatically identify patterns in large datasets, such as electronic health records and medical literature, to improve patient recruitment efficiency. Researchers have even developed a tool using large language models to rapidly review candidates and help predict patient eligibility, reducing patient screening time by over 40%.
3. Patient Accessibility and Limited Diversity
AI can play a critical role in improving access to clinical trials, especially for patients from underrepresented demographic groups. Inaccessibility and limited diversity contribute to low patient recruitment and retention rates and lead to inequitable drug development.
Clinical trial sites are generally clustered in urban areas and large academic centers, making access difficult for communities in rural or underserved areas. Financial burdens such as treatment costs, transportation, and childcare compound these barriers. These burdens are more pronounced in ethnic and racial minorities and groups with lower socioeconomic status.
As a result, racial and ethnic minority groups represent as little as 2% of patients in U.S. clinical trials, despite comprising 39% of the national population. This lack of diversity poses a significant risk, given that genetics vary across racial and ethnic populations and can influence adverse drug responses. Greater representation in clinical trials is essential to help researchers develop treatments that are both effective and safe for diverse populations, ensuring that medical advancements benefit everyone.
AI can help clinical trial sponsors by facilitating decentralized trials, which move trial activities to remote and alternative locations. Decentralized trials often use wearables to collect data digitally and AI-powered analytics to summarize relevant anonymized information. This hybrid approach can eliminate geographical barriers, making trials more accessible to a broader range of patients.
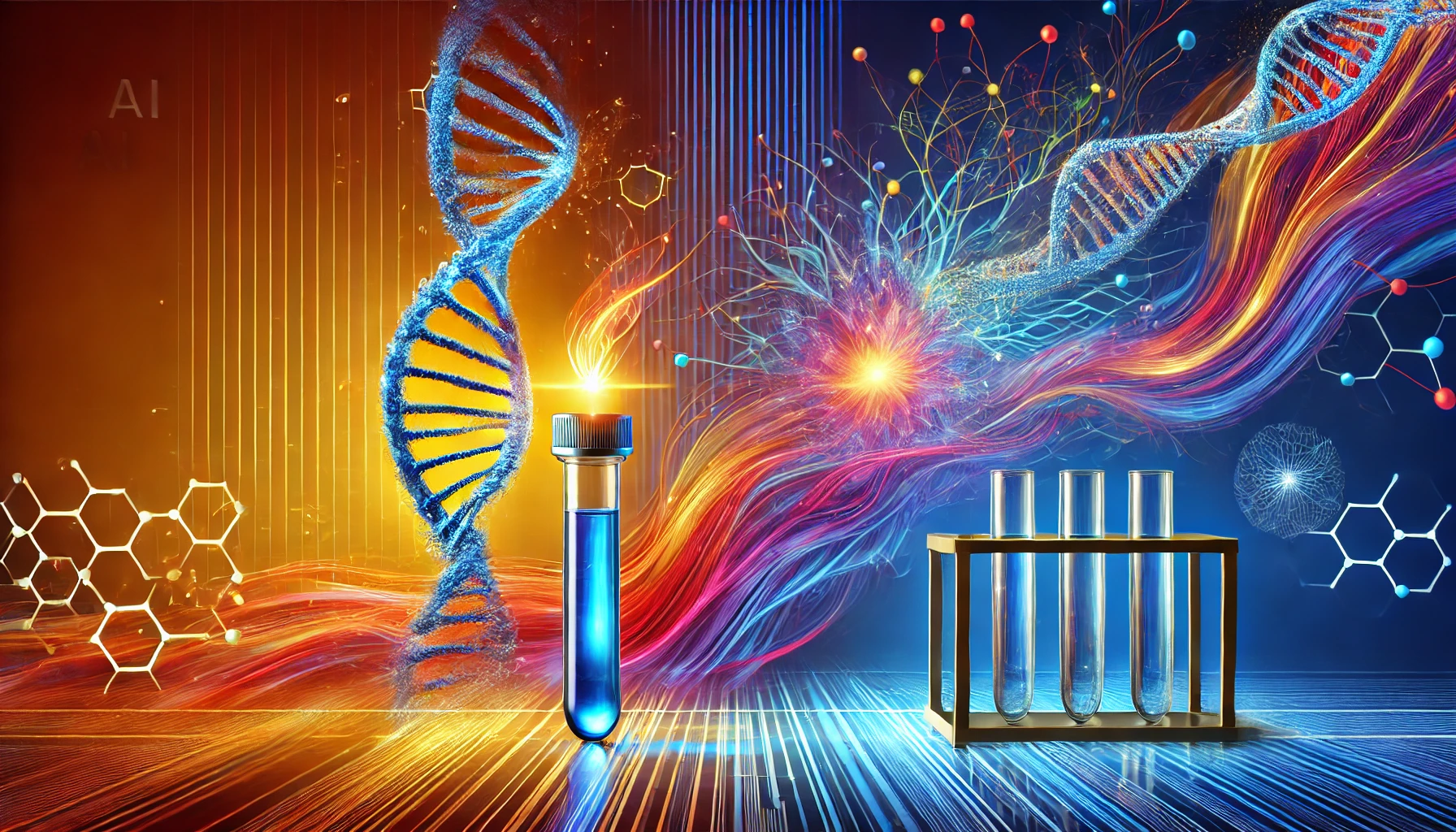
Smarter Trials Make Smarter Treatments
Clinical trials are poised to be transformed by AI. With its ability to analyze large datasets, identify patterns, and automate processes, AI can optimize trial design, enhance patient diversity, streamline recruitment and retention, and break down accessibility barriers. As the healthcare industry continues to adopt AI-powered solutions, the future of clinical trials has the potential to become more inclusive, patient-centered, and innovative. Embracing these technologies is about creating a clinical research ecosystem that accelerates drug development and delivers more equitable healthcare outcomes for all.