Microsoft Unveils BitNet b1.58 2B4T: A Breakthrough in Efficient AI Computing
Microsoft has introduced BitNet b1.58 2B4T, a groundbreaking large language model engineered for exceptional efficiency. Unlike conventional AI models that rely on 16- or 32-bit floating-point numbers, BitNet uses ternary quantization, representing each weight with just three discrete values: -1, 0, or +1. This innovative approach allows each weight to be stored in a mere 1.58 bits, significantly reducing memory usage and enabling the model to run on standard hardware without the need for high-end GPUs.
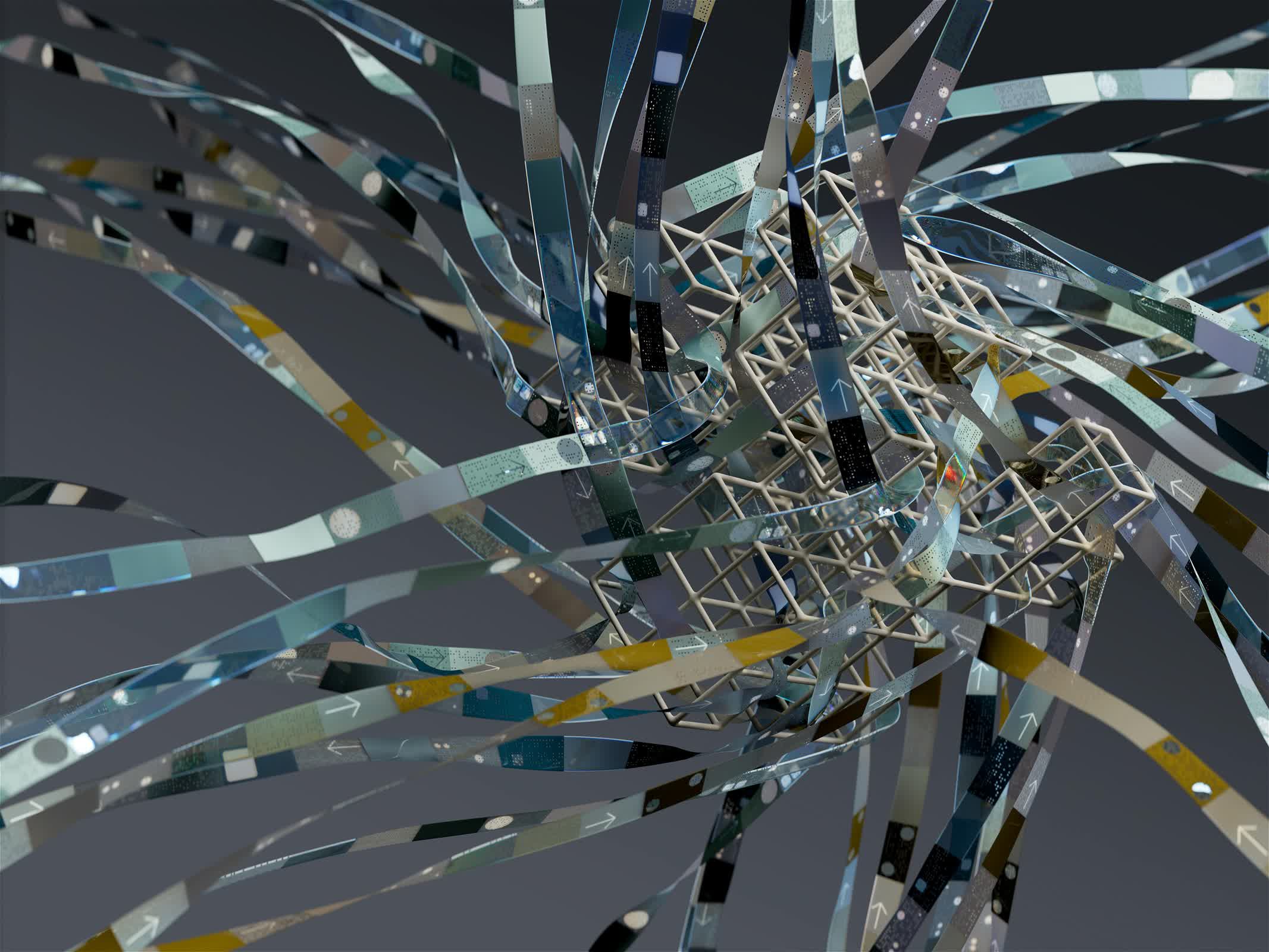
The BitNet b1.58 2B4T model, developed by Microsoft’s General Artificial Intelligence group, boasts two billion parameters and was trained on a massive dataset of four trillion tokens, equivalent to the contents of 33 million books. This extensive training enables BitNet to perform on par with, or even surpass, other leading models of similar size. In benchmark tests, the model demonstrated strong performance across various tasks, including grade-school math problems and questions requiring common sense reasoning.
Key Advantages of BitNet b1.58 2B4T
What sets BitNet apart is its remarkable memory efficiency. The model requires a mere 400MB of memory, less than a third of what comparable models typically need. This allows it to run smoothly on standard CPUs, including Apple’s M2 chip, without relying on specialized AI hardware. A custom software framework called bitnet.cpp, optimized for the model’s ternary weights, ensures fast and lightweight performance on everyday computing devices.
The development of BitNet b1.58 2B4T represents a significant shift in AI computing. By using extremely simple computations, mostly additions instead of multiplications, the model consumes far less energy. Microsoft researchers estimate that it uses 85 to 96 percent less energy than comparable full-precision models. This breakthrough could pave the way for running advanced AI directly on personal devices, eliminating the need for cloud-based supercomputers.
Future Prospects and Limitations
While BitNet b1.58 2B4T has shown impressive performance, it does have some limitations. The model currently supports only specific hardware and requires the custom bitnet.cpp framework. Its context window is also smaller than that of the most advanced models. Researchers are actively investigating why the model performs so well with its simplified architecture and are working to expand its capabilities, including support for more languages and longer text inputs.
The introduction of BitNet b1.58 2B4T marks a significant step forward in making AI more accessible and efficient. As research continues to refine this technology, we can expect to see even more innovative applications of AI in the future.