In Mental Health AI, Safety Isn’t Just Technical—It’s Deeply Human
In the field of mental health care, the integration of AI brings both excitement and skepticism. On one hand, there’s hope that smarter tools can scale access to care, reduce the burden on providers, and connect people to support faster. On the other hand, there’s caution because the personal nature of mental health care means the cost of errors isn’t measured in technical terms, but in human impact.
I’ve spent much of my career applying machine learning and AI in various contexts, and conversations with therapists, clients, and clinical teams have made it clear that the stakes in mental health are literally human lives. You’re not just optimizing for speed or personalization; you’re entering the most vulnerable spaces of someone’s life. Mental health care is built on trust, connection, and safety, and AI must become part of that dynamic purposefully.

At Spring Health, building trustworthy AI starts with designing for safety, consent, and equity from day one. This approach isn’t about abstract risk; it’s about real people in real moments looking for help, and the tools we build must meet that need with care.
What AI Safety Really Means in Mental Health AI
In most industries, AI safety focuses on technical accuracy, content moderation, and data protection. While these aspects are important in mental health care as well, the concept of “safety” is broader and more human-centric. It’s not just about whether a model performs well; it’s about whether someone feels respected, protected, and cared for in vulnerable moments.
To ground this work, we consider AI safety across four core pillars:
- Clinical Integrity: The first question we ask when building AI tools is whether they support the patient-provider relationship. Our AI tools are designed to support relational work, including space for reflection and human connection. Features like session summaries are built to reduce administrative burden, not to diagnose or direct care.
- Privacy and Consent: Privacy is foundational in mental health care. We secure data across every layer and embed transparency and consent into our products. Members and providers always know when AI is in use, and participation is always a choice.
- Governance: Trust is earned through clarity and accountability. Our AI governance board works together to evaluate risk, monitor behavior, and ensure responsible building.
- Model Fairness: Fairness is a continuous process of learning and improving. We invest in systems that monitor and surface patterns to our clinical and technical teams.
Spring Health’s Approach to AI Safety in Mental Health Care
Safety can’t be bolted on; it has to be built in from the first design conversation to the last line of code. We treat safety as a design principle, not a checklist. This mindset shows up across every part of the development process.
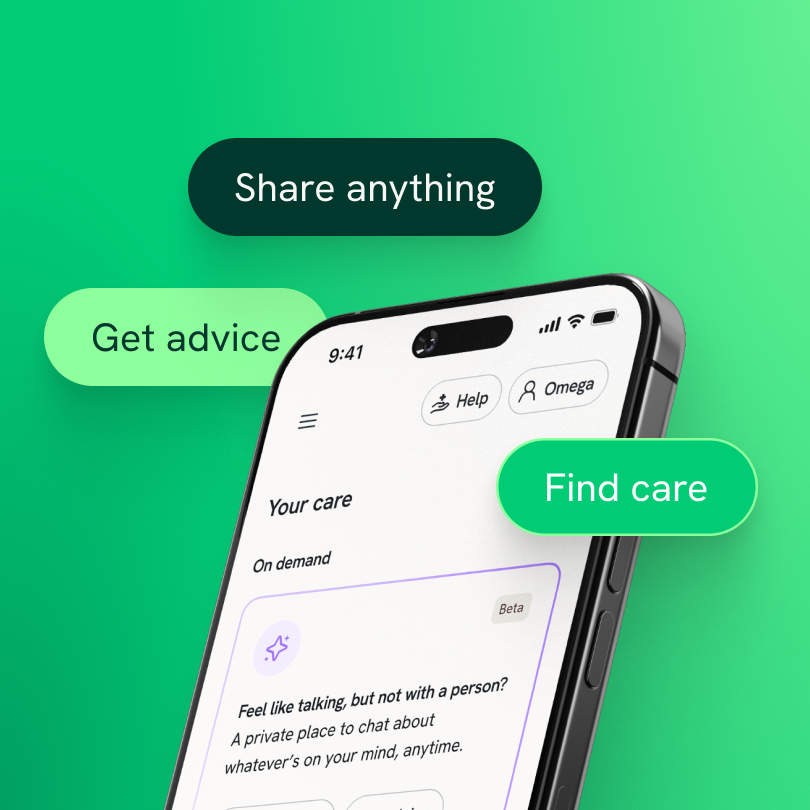
Our session summary tool, for example, routes output to the provider, not the member, giving providers space to review the content and decide how—or if—it should inform their next session.
Designing Mental Health AI Tools Clinicians and Members Trust
AI must be designed to function and earn the trust of providers and members. That means building systems that are transparent, consent-based, and always aligned with the clinical relationship. Our core principle is that AI is an enabler of care, not a replacement. AI can reduce the administrative weight that pulls clinicians away from their patients.
We see this across several tools, including pre-appointment intake, in-the-moment chat, and automated session summaries. Every interaction is opt-in, clearly labeled, and built to preserve the therapeutic alliance.
The Future of AI in Mental Health Care: Safe, Predictive, and Personalized
We’re building tools to improve access, safety, and connection without compromising trust. One opportunity is helping people find the right care faster. Our AI-powered intake and matching experiences are designed to reduce barriers to care.
We’re also investing in precision mental health care, using AI to support more personalized, responsive journeys. None of this matters if it isn’t safe, so we invest in bias evaluation, model monitoring, transparency standards, and human oversight.
.jpg)
Leadership in Mental Health AI Starts with Safety and Trust
AI in mental health isn’t a solved problem; it demands humility, responsibility, and constant iteration. At Spring Health, we’ve built AI not just to scale care but to protect and grow the relationships that power it. That means designing for safety, consent, and equity from the start.
To leaders reading this, our message is simple: Choose AI partners who don’t just use the technology but govern it. Ask the hard questions, look for nuance, and push for transparency. In this space, trust isn’t a buzzword; it’s the product.