Recent advancements in weather forecasting have led to the development of artificial intelligence (AI) based models, such as Pangu, which utilize deep neural networks trained on historical atmospheric data. These models have shown comparable or even superior forecast accuracy to traditional numerical weather prediction models. However, their performance in simulating certain atmospheric phenomena remains questionable.
This study examines the capability of the Pangu model to simulate mesoscale kinetic energy spectra by comparing it with ERA5 reanalysis data and simulations from the Model for Prediction Across Scales (MPAS). The analysis focuses on the upper troposphere and lower stratosphere, using pressure levels of 850 hPa, 300 hPa, and 100 hPa to represent different atmospheric layers.
Key Findings
- Predictive Ability: Pangu demonstrates superior short- to medium-term forecasting capabilities compared to MPAS models based on latitude-weighted root-mean-square error (RMSE) and anomaly correlation coefficient (ACC) evaluations.
- Kinetic Energy Spectra: While Pangu’s kinetic energy spectra agree with ERA5 and MPAS at large scales, it significantly underestimates mesoscale energy, particularly in the upper troposphere and lower stratosphere.
- Rotational and Divergent Kinetic Energy: The difference in kinetic energy spectra between Pangu and ERA5 is dominated by rotational kinetic energy in the upper troposphere and divergent kinetic energy in the lower stratosphere.
- Spectral Budget Analysis: Pangu and MPAS exhibit stronger upscale energy cascades than ERA5 at large scales, primarily due to stronger baroclinic instability. However, Pangu underestimates the downscale energy cascade at mesoscales.
- Vertical Motion: Diagnosed pressure vertical velocity shows that Pangu underestimates vertical motion at mesoscales, which is crucial for accurately simulating mesoscale energy.
Conclusion
While Pangu achieves high prediction accuracy in short- to medium-term forecasts, its inability to reasonably simulate mesoscale energy limits its reliability for predicting rapidly developing extreme weather systems. The study highlights the need for AI-based models to improve their representation of mesoscale processes and vertical motion. Future work should explore additional model configurations and observational datasets to further validate these findings.
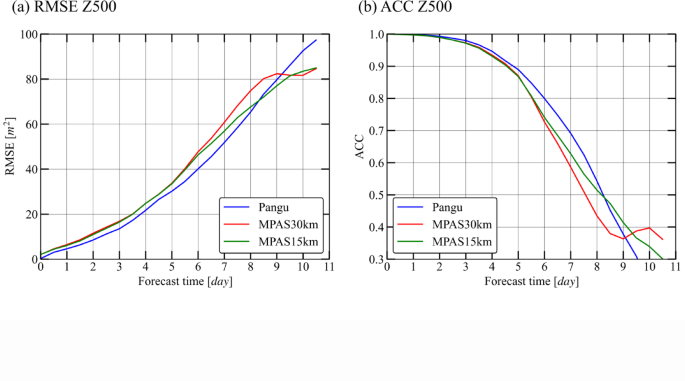
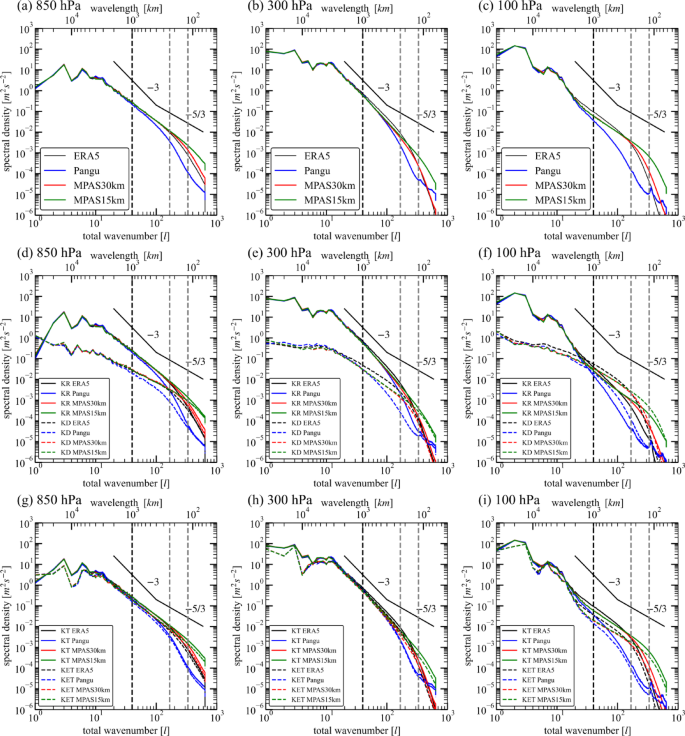
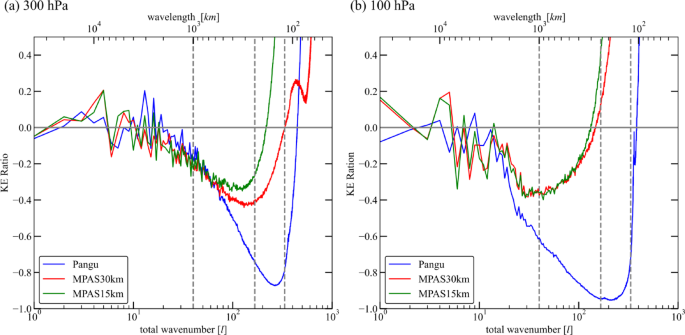
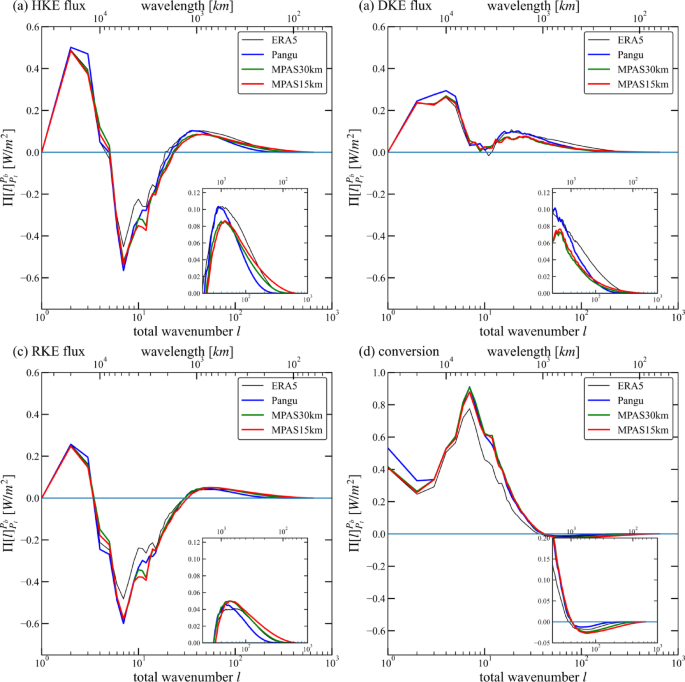
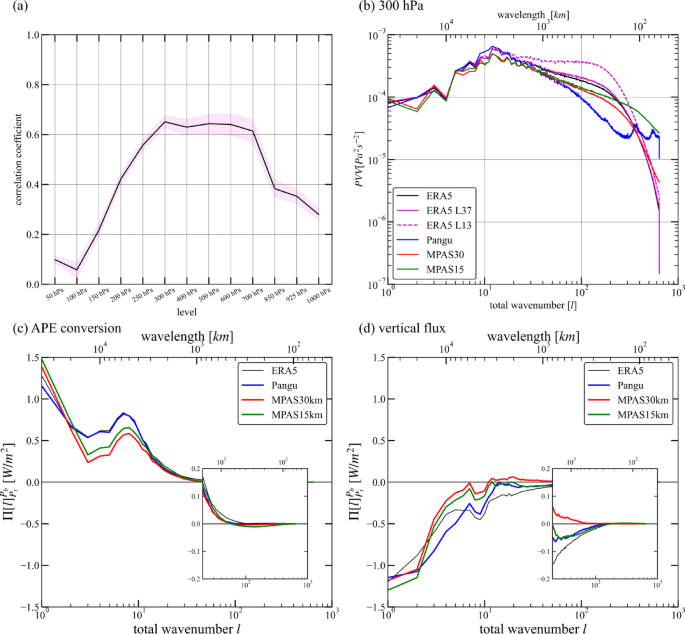