Google’s recent comparisons between their Ironwood TPU v7p pod and the El Capitan supercomputer at Lawrence Livermore National Laboratory have sparked controversy due to their misleading nature. The comparison between these two vastly different systems – one designed for AI workloads and the other for traditional HPC simulations – is not inherently problematic, but Google’s execution was flawed.
The Misleading Comparison
Google compared the theoretical peak performance of an Ironwood pod with 9,216 TPU v7p compute engines to the sustained performance of El Capitan running the High Performance LINPACK (HPL) benchmark at 64-bit floating point precision. This comparison is considered ‘silly’ as it pits the peak performance of one system against the sustained performance of another at different precision levels.
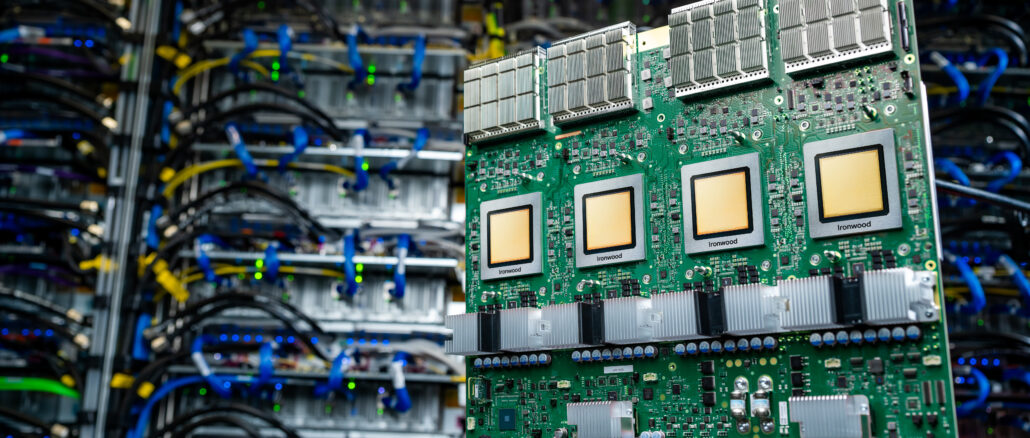
Cost and Performance Analysis
A more meaningful analysis involves considering both performance and cost. The article provides a detailed comparison of various AI and HPC systems, including their costs and performance at different precision levels. Key findings include:
- The estimated cost of an Ironwood TPU v7p pod is around $445 million to build and over $1.1 billion to rent over three years.
- The El Capitan supercomputer, built by Hewlett Packard Enterprise, cost $600 million and provides better performance per dollar at FP16 resolution.
- The Aurora machine at Argonne National Laboratory, with a final cost of $200 million after Intel’s $300 million write-off, offers the best performance per dollar at $12 per teraflops for FP16 precision.
Detailed Performance Comparison
- Ironwood TPU v7p pod: 21.26 exaflops at FP16, 42.52 exaflops at FP8
- El Capitan: 2.05 times more performance than Ironwood at FP16/FP8, with 2.73 exaflops peak at FP64
- Cost per teraflop: $21 for Google to use Ironwood, $52 to rent it, compared to $14 for El Capitan and $12 for Aurora at FP16
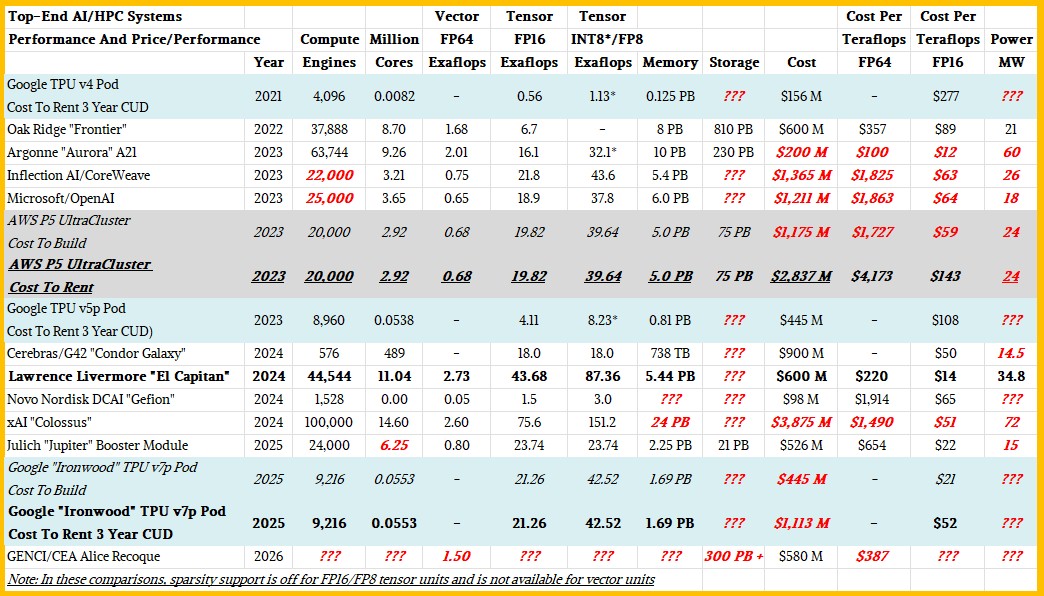
The analysis concludes that while Google’s Ironwood pod is a powerful AI system, comparisons to traditional HPC machines like El Capitan must be made carefully and with consideration of both performance and cost factors. The cost of capability-class AI supercomputers has risen to billions of dollars, making cost-effectiveness a critical consideration.