Machine Learning and War Termination: Using AI to Explore Peace Scenarios in Ukraine
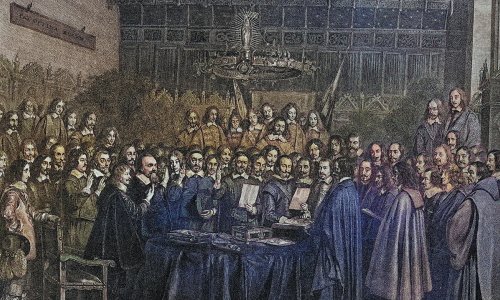
Talks aimed at ending the war in Ukraine are underway, bringing with them a complex set of challenges and opportunities. While the urgency for a ceasefire is apparent, historical precedent suggests that peace negotiations often extend beyond initial expectations. Understanding the factors influencing war termination, including the role of artificial intelligence (AI), is crucial for navigating the path toward a negotiated settlement. This report, from the Center for Strategic and International Studies (CSIS), explores the ways AI can be applied to examine potential peace scenarios.
The Complexities of War Termination
War termination is inherently complex, shaped by numerous factors beyond battlefield successes. Often, a negotiated settlement presents a viable option. A CSIS report, using data from the Uppsala Conflict Data Program (UCDP), illustrates that a majority of wars conclude through compromise. This underscores the importance of identifying the critical influences on the process of ending conflict. These factors can vary significantly depending on the specific circumstances of each conflict.
Leveraging AI for Analysis
Artificial intelligence can help navigate the complexities of war termination. Large language models (LLMs), while generalists, can be refined using techniques like retrieval-augmented generation (RAG), few-shot learning, and chain-of-thought reasoning. The effectiveness of AI relies not only on the model itself but also on the data quality and the structure of the questions used. By analyzing datasets focused on historical peace negotiations and structuring prompts to reflect real-world decision-making, AI can go beyond summarization to systematically analyze war termination processes.
This study employed AI to explore potential pathways to peace between Russia and Ukraine. Researchers at the CSIS Futures Lab applied existing literature on peace negotiations in international relations (IR) to curate datasets and refine their approach to prompt engineering, incorporating techniques such as few-shot learning, in-context learning, and chain-of-thought reasoning. The study proves that AI techniques like RAG can be used to quickly understand and summarize key documents related to a potential peace agreement in Ukraine.
Key Recommendations for Policymakers
AI-driven analysis offers key recommendations for policymakers assessing possible pathways to peace. These include:
- Territorial control and security guarantees: These will be central to any agreement. Both the AI-driven analysis and recent peace discussions highlight the significance of these factors.
- Transparent, fair, and inclusive negotiation process: A lasting peace requires one that fully engages Ukraine and European states. This stands in contrast to proposals that prioritize negotiations between Moscow and Washington while pressuring Ukraine into concessions.
- Clear trade-offs: Negotiators must identify trade-offs, recognizing that political realities will ultimately dictate what is negotiable. Leverage remains in economic reparations.
- Sustained international involvement: A lasting peace will require sustained international involvement, including mediation, security guarantees, and enforcement mechanisms.
- Responsible use of AI: Generative AI is a powerful tool but requires careful use. AI should support, but not replace expert human judgment.
What Do We Know About War Termination and Peace Processes?
IR scholars focus on how wars start and end. Bargaining theory suggests conflicts end when the perceived benefits of peace outweigh the costs of continued fighting. Information plays a critical role, influencing negotiation outcomes. Domestic political factors, including shifts in coalitions, also shape negotiations, as can domestic “spoilers.”
Durable peace agreements often include civil society actors, such as local religious organizations or human rights groups. Additionally, some form of justice, such as post-conflict trials or reparations, can contribute to the durability of peace agreements. The international community also plays a role through mediation efforts, monitoring, and providing security guarantees.
Applying AI to Analyze War and Peace
To address the complex question of a potential peace agreement, the CSIS Futures Lab leveraged data sources, the Scale AI’s Donovan platform, and a data query method known as RAG.
RAG enhances LLM performance by directing models to leverage specific data sources when generating outputs. This relies on a “retriever” and a “generator.” Other techniques like “few-shot learning” and “chain-of-thought prompting” enhance LLM outputs. The research team incorporated these elements.
The data included:
- Full text of peace agreements from the UCDP.
- Published articles curated by CSIS scholars, analyzing potential peace talks.
The research team’s analysis included a prompting strategy designed to guide the LLM’s analysis.
Key Negotiation Factors and Challenges in the Case of Ukraine and Russia
Effectively employing AI requires gathering the right data and developing a tailored prompting approach.
The figure below illustrates the prompting strategy.
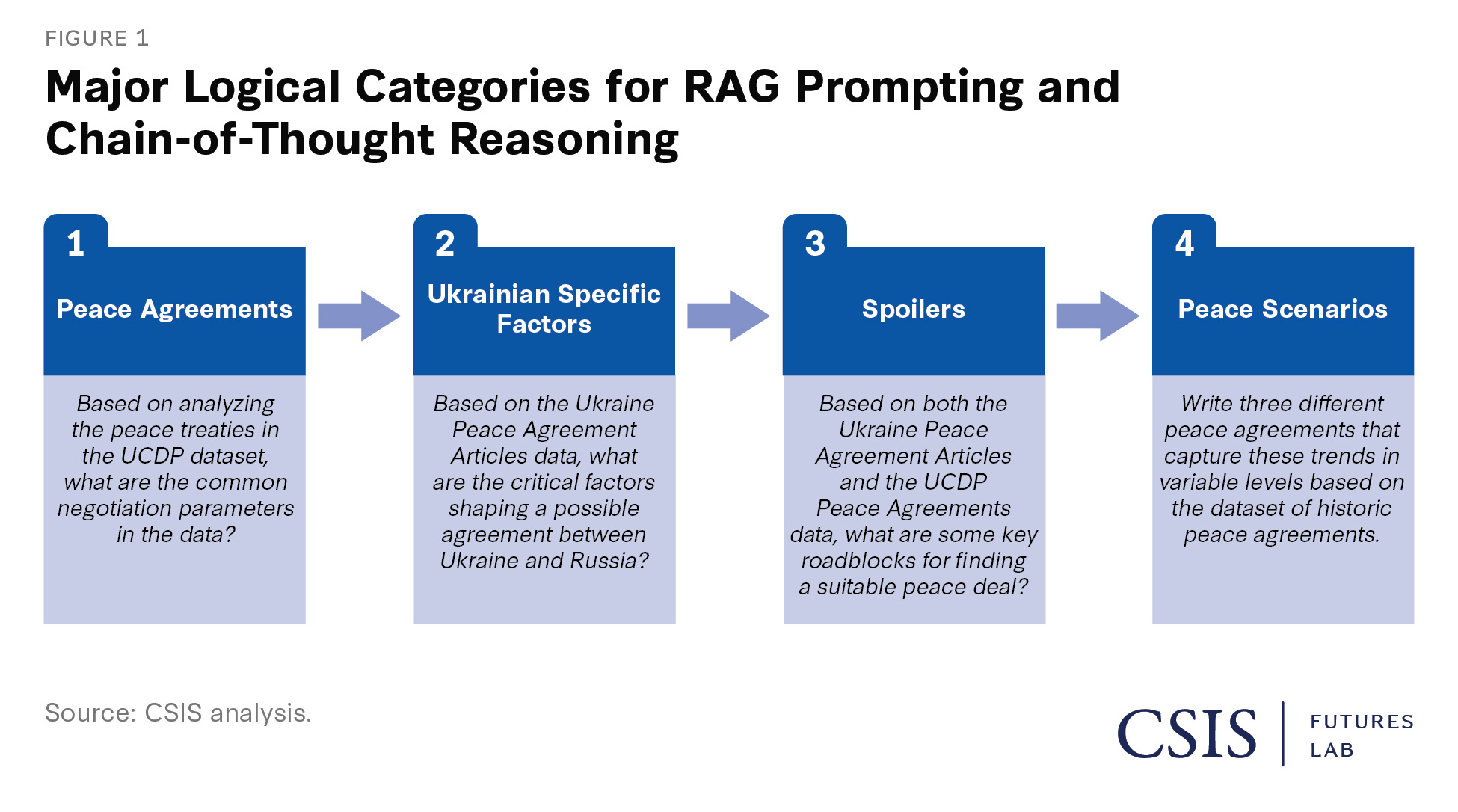
The AI analysis identified common negotiation parameters, including:
- Clear agenda and methodology for negotiations.
- Timelines and processes for conducting negotiations.
- Security guarantees and shared principles.
- Monitoring and evaluation mechanisms.
- Economic factors and social welfare issues.
The AI also helped identify potential roadblocks, such as power imbalances and divergent views on territorial control.
From AI to Policy: Parameters of a Future Deal
AI-driven modeling identified several core takeaways for policymakers:
- Territorial control and security guarantees will define any deal.
- Political realities will constrain peace terms.
- The international community will determine whether peace holds.
- AI can support negotiations, but it won’t replace strategic judgment.
Generative AI provides valuable insights for peace settlements when used with expert judgment. Best practices include using AI for comparative analysis, applying structured prompting, incorporating expert validation, customizing data inputs, and leveraging AI for scenario planning. AI is a tool for analysis, not a substitute for political decision-making.
The war’s changing nature presents challenges that AI alone cannot resolve. AI struggles to adapt to rapid shifts in political dynamics. Policymakers must avoid treating AI as a shortcut to diplomacy. AI can help structure negotiations, but it can’t replace strategy.
This analysis suggests that AI tools do not serve as a replacement for human judgment, expertise, and creativity. To make AI useful, policymakers must treat it as a force multiplier, not a decision-maker.
About the Authors
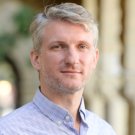
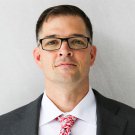