Today’s AI systems are rapidly improving and could soon bridge the gap with human capabilities on complex tasks, according to a new analysis. A non-profit organization, METR, created a metric to assess the progress of AI models, providing a clearer picture of their advancements.
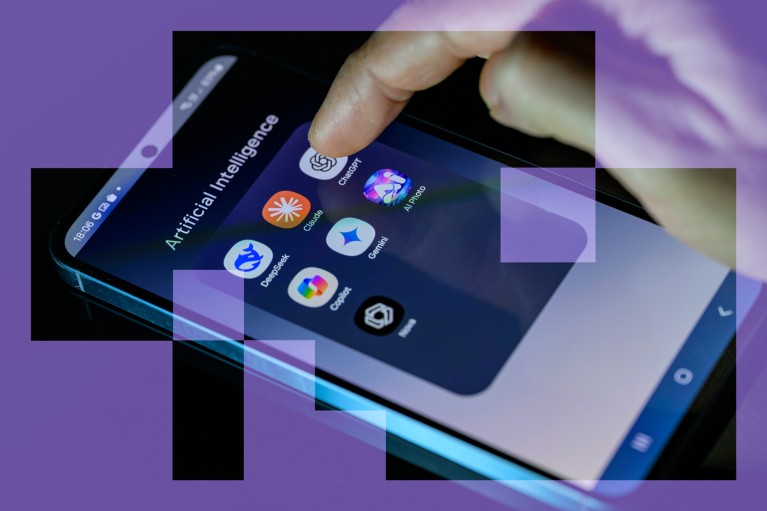
METR, based in Berkeley, California, developed nearly 170 real-world tasks across coding, cybersecurity, general reasoning, and machine learning. They established a ‘human baseline’ by measuring the time expert programmers took to complete these tasks. The team’s new metric, called ‘task-completion time horizon,’ assesses how long it takes programmers to complete tasks with the same success rate as AI models.
In a recent preprint on arXiv, METR reported that GPT-2, an early large language model (LLM) from OpenAI (2019), failed on all tasks that took human experts over a minute. In contrast, Anthropic’s Claude 3.7 Sonnet, released earlier this year, completed half the tasks that would take humans 59 minutes. The study found that the time horizon of the 13 leading AI models has roughly doubled every seven months since 2019. Notably, this growth accelerated in 2024, with the latest models improving at a rate of doubling every three months.
METR suggests that AI models could handle tasks taking humans about a month at 50% reliability by 2029, possibly sooner, based on the current progress. One month of dedicated human expertise, the paper notes, can be enough to start a new company or make scientific discoveries, for instance.
Professor Joshua Gans of the University of Toronto, known for his work on the economics of AI, cautioned against putting too much weight on these types of predictions. “Extrapolations are tempting to do, but there is still so much we don’t know about how AI will actually be used for these to be meaningful,” he said.
Co-author Lawrence Chan explained that the 50% success rate was chosen because it was the most robust to slight variations in data. Raising the threshold to 80% significantly reduced the average time horizon, although the overall growth trends remained similar.
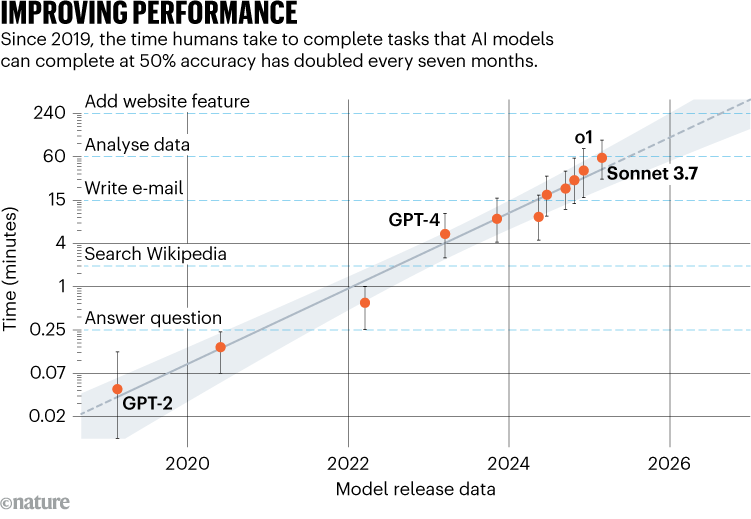
Improvements over the past five years in the general capabilities of LLMs are largely tied to increases in scale, encompassing data, training time, and model parameters. The paper credits advancements in the time horizon metric mainly to AI model improvements in logical reasoning, tool use, error correction, and self-awareness in task execution.
METR’s approach addresses the limitations of existing AI benchmarks that loosely map to real-world work and quickly plateau as models improve. Co-author Ben West noted that this new method provides a continuous measure that better captures meaningful progress. He also pointed out that despite achieving superhuman performance on many benchmarks, leading AI models haven’t yet had a significant economic impact, because the best models currently have a time horizon of around 40 minutes. However, Anton Troynikov, an AI researcher and entrepreneur in San Francisco, suggests that AI would have more economic impact if organizations were more willing to experiment and invest in leveraging the models effectively.