Nvidia Charts a Course for AI Leadership Across Industries
If Nvidia hopes to lead the charge in the rapidly evolving world of artificial intelligence, from hardware and software to developer tools, it must adapt to the myriad environments where this technology is taking root. That was the message from Nvidia co-founder and CEO Jensen Huang during his keynote address at the GPU Technology Conference (GTC) 2025 in San Jose this week.
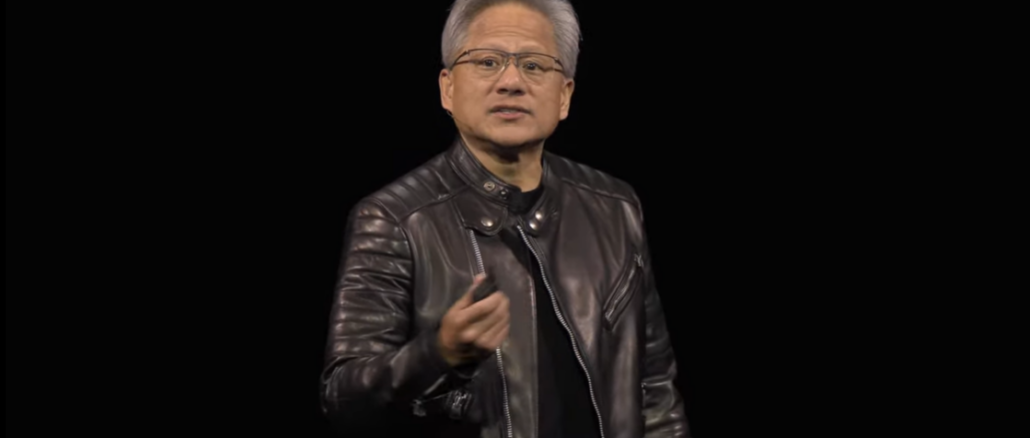
Beyond discussing Nvidia’s “Blackwell” B300 GPUs and the upcoming “Rubin” accelerators, Huang highlighted the company’s focus on enterprise applications, edge computing, and the emerging field of physical AI, emphasizing Nvidia’s commitment to providing solutions for all these areas.
“Cloud service providers, of course, like our leading-edge technology,” Huang explained. “They like the fact that we have a full stack, because accelerated computing is not about the chip… It’s the chip, the programming model, and a whole bunch of software that goes on top of it. That entire stack is incredibly complex.” He added that the fact that Nvidia CUDA developers are also CSP customers further strengthens the ecosystem, as they are building fundamental infrastructure for the world.
However, Huang pointed out that while AI gained its initial momentum in the cloud, its application extends far beyond.
“Now that we’re going to take AI out to the rest of the world… AI, as it translates to enterprise IT, as it translates to manufacturing, as it translates to robotics and self-driving cars, or even companies that are starting GPU clouds, they have their own requirements,” Huang said. He noted how AI and machine learning are reinventing the computing stack, from processors to applications, and the need for enterprises to adapt to new ways of running and orchestrating these resources.
Rather than simply retrieving and reading data, enterprise users will increasingly pose questions to AI systems for direct answers. “This is the way enterprise IT will work in the future,” Huang predicted. “We’ll have AI agents, which will be part of our digital workforce… AI agents will be everywhere. How they run, what enterprises run, and how we run them will be fundamentally different. So we need a new line of computers.”
This shift begins with new hardware, including two personal AI supercomputers: the DGX Spark (formerly Project DIGITS) and the DGX Station. These are Blackwell-powered desktop systems designed for inference and other tasks, perfect for local use or operation within Nvidia’s DGX Cloud or other accelerated cloud environments.
The DGX Spark is equipped with a GB10 Grace Blackwell Superchip, designed to deliver up to a thousand-trillion operations per second for AI fine-tuning and inference. The DGX Station boasts the GB300 Grace-Blackwell Ultra Desktop Superchip and features 784 GB of coherent memory, Nvidia’s ConnectX-8 SuperNIC, the AI Enterprise software platform, and access to the vendor’s NIM AI microservices.
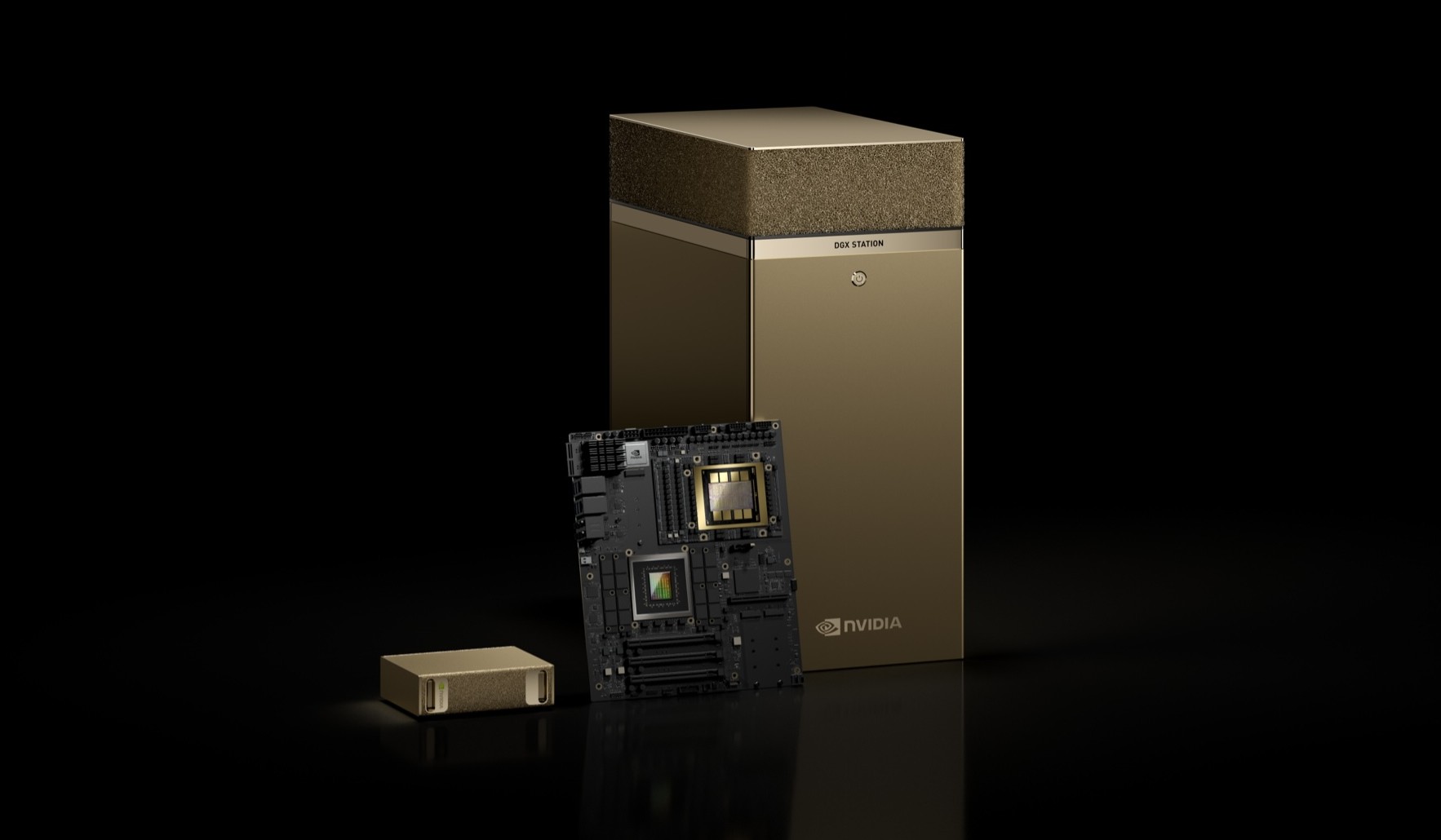
These systems offer enterprise users new tools for AI workloads and also provide a stepping stone into the era of AI reasoning models. This innovative approach surpasses the capabilities of AI agents, allowing them to address and solve detailed problems in ways that go far beyond the simple prompt-and-reply mechanics commonly associated with current chatbots.
“We now have Ais that can reason, which is fundamentally about breaking down a problem, step by step,” Huang stated. “Now we have Ais that can reason step by step by step using … technologies called chain of thought, best of N, consistency checking, path planning, a variety of different techniques. We now have Ais that can reason.”
At the Consumer Electronics Show in January, Nvidia unveiled its Llama Nemotron advanced agentic AI models and Cosmos Nemotron vision language models. These models are now available as NIM microservices, allowing developers to build AI agents capable of understanding language and the world, and responding accordingly.
At GTC, Nvidia revealed a family of open Llama Nemotron models with enhanced reasoning capabilities for multistep math, coding, decision-making, and instruction following. According to Kari Briski, vice president of generative AI software for the enterprise at Nvidia, the company also is supplying 60 billion tokens of its artificially generated synthetic datasets to further assist developers in adopting these models.
“Just like humans, agents need to understand context to breakdown complex requests, understand the user’s intent, and adapt in real time,” Briski said. The Nemotron models, which come in three sizes – Nano, Super, and Ultra – allow users to toggle the reasoning capability on and off. The Nano model is the smallest and offers high accuracy on PCs and edge devices, while the Super model delivers high accuracy and throughput on a single GPU. Ultra models will run on multiple GPUs. The Nano and Super models are currently available, with Ultra coming soon.
Another noteworthy addition to Nvidia’s AI Enterprise software platform is AI-Q Blueprint, a NIM-based offering that allows enterprises to connect proprietary data to reasoning AI agents. This software integrates with Nvidia’s NeMo Retriever tool to query various data types—including text, images, and videos. It allows accelerated computing to work with third-party storage platforms and software.
“For teams of connected agents, the blueprint provides observability and transparency into agent activity, allowing the developers to improve agents over time,” Briski added. “Developers can improve agent accuracy and reduce the completion of these tasks from hours to minutes.”
Nvidia’s AI Data Platform—a reference design for enterprise infrastructure—incorporates AI query agents built using the AI-Q Blueprint.
In his keynote, Huang emphasized physical AI, an area that involves integrating AI into physical systems to enable them to perceive and react to the real world. He believes this space potentially could become the largest in the AI market.
“AI that understands the physical world, things like friction and inertia, cause and effect, object permanence, that ability to understand the physical world, the three-dimensional world… It’s what’s going to enable a new era of physical AI and it’s going to enable robotics,” Huang explained.
Nvidia has made several announcements in this area, including its new AI Dataset designed for robotics and autonomous vehicles. Developers can use this dataset for pretraining, testing, and validation of models, or for fine-tuning foundation models after training. It features real-world and synthetic data used for the Cosmos world model development platform, in addition to the Drive AV software, Isaac AI robot development platform, and Metropolis framework for smart cities.
The first iteration of the dataset is available on Hugging Face, offering 15 terabytes of data for robotics training, with support for autonomous vehicle development soon to come.
Huang also highlighted Nvidia’s Isaac GROOT N1. This is an open foundation model trained on real-world and synthetic data, designed for humanoid robots. It is a result of Project GROOT, the vendor first unveiled at last year’s GTC.